9 Awesome Examples of Value Created Through Deep Learning & AI
Over time, human beings have invested exquisite quantities of sources in evolving and perfecting AI. The intention being transformation that yields most effective performance for a couple of industries and applications.
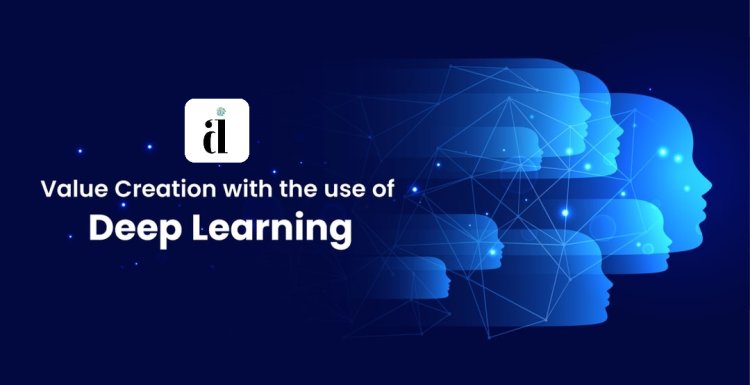
We stay withinside the age of wonderful technological advancements. With each passing day, the human race ticks off some other container on its checklist. One that for eons could have regarded unimaginable. The pioneer of this quest being the sunrise of Artificial Intelligence.
Over time, human beings have invested exquisite quantities of sources in evolving and perfecting AI. The intention being transformation that yields most effective performance for a couple of industries and applications.
In this article, we pass over one such subset of AI- Deep Learning. Let`s decode what deep gaining knowledge of is, the way it works, and real-existence examples of deep gaining knowledge of in action.
- Deep Learning is a sub-department of Machine Learning, a shape of Artificial Intelligence. Its innovation has helped conquer boundaries of Machine Learning and made AI relevant to a broader set of use cases. Let's begin on the very pinnacle and primary recognize Artificial Intelligence.
What is Artificial Intelligence or AI?
Artificial Intelligence bases itself at the concept that human choices are mathematical computations. This means that it's far feasible to teach machines with algorithms and get the identical conclusions a human would.
Evolution of AI
The idea of a humanoid or machine capable of thinking like a human has been around for centuries. He continued to appear in works of myth, legend and fiction. From Talos in Greek mythology to golems in Jewish folklore, people have been interested in AI since time immemorial.
AI was founded in 1956 as an academic discipline. Scientists from various fields have worked hard to create artificial brains.
Over the years, AI has garnered supporters and skeptics alike. The unremitting efforts of those committed to this idea gradually led to a consensus on the usefulness of AI.
The first such monumental achievement is Deep Blue. It was the first computerized chess system manufactured by IBM. On May 11, 1997, Deep Blue defeated current world champion Garry Kasparov. It was made possible thanks to its ability to process 200,000,000 movements per second.
AI in the 21st Century
The twenty first Century got here ready with advanced laptop systems, get entry to to massive statistics, and optimism closer to AI. This fueled the big reputation and alertness of diverse AI gear throughout industries.
AI mania took over the markets and made its location withinside the fields of ecology, economics, or even client merchandise. Since then, speedy improvements are being made thru studies and development.
The international marketplace for AI hardware, software, services, and era is forecasted to develop from USD 58.three billion in 2021 to USD 309.6 billion with the aid of using 2026. There has been good sized development in textual content analysis, picture and video processing, or even speech recognition.
AI-primarily based totally gear have made their manner into our everyday lives today. While we understand a few as AI, a few are greater cloaked. Banking software, statistics mining, or even Google`s Search engine are the various noteworthy achievements of AI. However, they regularly get decreased to simply merchandise of evolving laptop science.
What is Machine Learning or ML?
Machine learning is a kind of artificial intelligence. Machine learning algorithms analyze large amounts of data to identify patterns. This analysis allows the algorithm to predict outcomes based on past behavior.
The Evolution of Machine Learning
For many years, machine learning has been part of the artificial intelligence curriculum. However, by the late 1970s, AI focused on knowledge-based approaches and abandoned algorithms. This created a rift between the two disciplines.
Engineers and researchers in this area have been reorganized into separate areas. Now the focus has been on solving everyday real-life problems.
21st Century Machine Learning
The advent of the Internet has made data more accessible. In the 1990s, ML was able to prove its usefulness to a wider audience and thrive.
Since then, machine learning applications have provided solutions to common industry problems. Sales data analysis, product recommendations, and dynamic pricing have helped increase the company's resilience. Speech recognition, facial recognition and fraud detection have made our systems more secure.
Machine Learning Algorithms are more common than ever. Facebook feeds, Netflix recommendations, and even stock predictions are powered by machine learning with deep learning.
What is Deep Learning?
Deep learning is a type of machine learning. Efforts to replicate the neural networks of the human brain form the backbone of the DL algorithm. Advances in deep learning enable machines to make much more complex predictions than ever before. It also allowed for higher precision than before.
As the name suggests, deep learning is much deeper and more multi-layered than machine learning. He refutes linear learning and adapts to more complex processes. As deep learning advances, the goal remains to achieve high-level and accurate inference using raw input.
What Are Neural Networks?
Neural networks are the foundation upon which deep learning algorithms operate. Designed to mimic how the human brain works, these devices form a multi-layered network.
Neurons make up the different layers of a neural network. These interconnected neurons facilitate the transfer of information. Neural network layers can be broadly divided into three types.
Input Layer
The input data is first divided into pixels. Then each pixel is assigned to a neuron in the input layer. The channel then passes this information to the next level. They also determine which neurons should fire in the next layer.
Hidden Layers
The process of analysis and transmission through channels continues through several hidden layers. Selected neurons are executed at each step to ensure correct output. Bias (the number assigned to neurons) and channel weights are constantly adjusted. It also differs between layers and algorithms for determining the resulting output.
Output Layer
At the other end of the network is an output layer. Data transmitted and analyzed between the input layer and the hidden layer is exposed through the output layer.
Types of Deep Learning
Deep learning or machine learning can be done in a number of ways. The path you choose determines how the algorithm analyzes the data, the amount of human intervention required, and the final outcome. There are two main types of training.
Supervised Learning (SL)
In this way, the variables are well labeled. This means that the input has already been marked as the correct output. The machine learns to mark them together.
Supervised learning, as the name suggests, is similar to supervised learning in which students get the correct answers.
This method is suitable for simple tasks. We start by creating a well-known training data set. At the end of the training process, you can test your data. A subset of the training data is the backbone of a test to determine the correctness of the predicted output. [Infographics: Named Process Forms, Learning, Testing, Results] The SL model works in real world applications such as fraud detection, spam filtering, risk assessment or social media algorithms. This is the most commonly used of the three types.
Learning without a teacher
Unlike supervised learning, inputs in unsupervised learning are not flagged. Instead, training occurs on unlabeled datasets. Patterns are identified without hints. Learning without a teacher is similar to how the human brain processes information.
The purpose of unsupervised learning is to analyze the initial data to find basic similarities and to group the data based on them. Without labels, complex and complex processing is possible. This opens the range of data types that can be analyzed.
9 Applications and Examples of Deep Learning with Examples
1. Entertainment
Deep learning has helped create, publish and deliver entertainment media. Analyzing human body language with a camera makes modeling virtual characters easy. Deep video analytics accelerated the editing process, audio-video synchronization, and transcription. Deep learning has revolutionized filmmaking.
Streaming services and social media platforms use deep learning. This helps to provide a more personalized experience for end users. Deep learning provides the best targeting from recommendations to advertisements. Netflix, Amazon, YouTube, and Facebook include deep learning in their algorithms.
Sports Entertainment also benefits from DL. Analysis of player emotions, audience reactions, and more. It helps you pick the best moments from hours of footage. IBM Watson at Wimbledon 2018 is a good example of this.
4 Without labels, complex and confusing processing is possible. This opens a range of data types that can be analyzed.
2. Virtual Assistant
Today, virtual assistants have the same abilities as humans, depending on your will. You can also take notes, perform tasks, and suggest suggestions for voice commands. The virtual assistant uses deep learning to extract data. We all know our voices, our accents, the places we visit, even the songs we love. This will help them get better over time and better adapt to your needs. Deep Learning is at the heart of Siri, Alexa, Google Assistant and most other virtual assistants.
3. Visual Perception
Visual recognition systems range from basic to multi-layered. Deep learning models can identify and sort images based on location, objects, and even people.
Analysis of obscene images on social media helps create a safer environment for all. Visual recognition gives you access to the images you want from a vast library of search engines. You can also sort images in the gallery to quickly find what you need.
Facial Recognition has been used in security applications for many years. Now your smartphone is also unlocked with facial recognition. All of these revolutions are happening thanks to deep learning.
4. Health
The healthcare industry is a prime example of how deep learning has helped improve people's lives. Over the years, GPU-based systems have simplified the work of healthcare professionals. It has also contributed to effective diagnosis, standardized treatment, and improved overall performance.
Deep Learning-Based Medical System
- Addressing the shortage of quality manpower
- Accurate early diagnosis
- Provides the best pathology reports
- Predicting an outbreak or epidemic
- Treatment route standardization
- Development of new drugs and vaccines
Although deep learning often faces skepticism, it is increasingly being used for research purposes. Many healthcare giants are also adopting deep learning models to deliver faster, better-quality care and lower costs.
5. Natural Language Processing (NLP)
Natural language processing, or NLP, is the analysis and understanding of text or speech to get the right results. The complexity and nuances of human language are endless. So you benefit from systems like deep learning that learn and improve as you progress. The
NLP is adopted when summarizing long reading materials such as legal documents. It also helps with text classification, sentiment analysis, and answering questions. Customer service and chat tools are also suitable for NLP. The ability to understand complexity and construct its own syntax allows bots to operate with unparalleled efficiency.
6. Fraud Detection
The banking and financial sector is no stranger to scams and scams. Implementation of a deep learning-based security system has helped add an extra layer of protection. The model detects patterns in customer transactions, tracks credit ratings, and raises alerts for unusual activity. This implementation prevented credit card fraud and saved on recovery and insurance costs.
7. Language Translation
As the world gets smaller, the need to translate information increases. Deep learning allowed the software to identify characters and translate them into the desired language. Automatic machine translation can currently be done in two ways: automatic text translation and image translation.
These tools are useful not only for global business purposes, but also for everyday life. Whether you are a tourist or want to make a special gesture to your friends abroad, language is no longer an obstacle. This is all thanks to apps like Google Translate, Google Lens, and more. This includes deep learning to bridge the gap.
8. Pixel Recovery
For many years, the quality of smartphone cameras and security systems was still an issue. In many cases, it remains the same. Enlarging a video to identify a person is often difficult due to limited resolution. In 2017, a Pixel Recursive Super Resolution DL network trained by Google Brain researchers found a solution.
The network was able to capture and enhance low-resolution facial images. The improvements were significant enough to highlight features and enable identification. There are many image enhancement applications using deep learning. But most importantly, it can be used by police departments and law enforcement agencies to strengthen the judicial system.
9. Self-driving car
Ideas that once only appeared in fantasy dreams are now an unimaginable reality. What fuels this impressive feat in which people are approaching perfection? You guessed it, deep learning. A layered network of deep learning algorithms brings self-driving cars to life. Self-driving cars can identify signs and routes, manipulate traffic conditions, and even take into account real-time factors such as traffic congestion.
This is possible with data from cameras, sensors and mappings. Research continues to refine deep learning models to create the ultimate autonomous vehicle. The autonomous vehicle is designed to solve many of the problems humans face on a daily basis. It can be used in many occasions, as well as everyday shipping and commercial shipping.
Market Response to Deep Learning
Deep learning started solving real-world problems with original solutions. It would not be an exaggeration to say that the goal has been achieved.
The adoption and implementation of deep learning is impacting new industries every day.
Let's take a look at some important market factors that give us a glimpse into DL's promising future.
- During the forecast period 2020 to 2025, the Deep Learning market is expected to register a CAGR of 42.56%.
- As of 2019, North America has the largest share of the DL market.
- The Oceania subregion and the Indo-Pacific region are the fastest growing deep learning markets.
- Important players include Facebook Inc., Google LLC, Microsoft Corporation, IBM Corporation, and Amazon Web Services Inc.
Limitations of Deep Learning
Given the revolutionary power of deep learning, pointing out its limitations may seem unreasonable. However, it is important to view the constraints as these constraints. They mean nothing more than room for improvement and growth.
Let's take a look at the current limitations of deep learning as we know it.
1. Huge amount of data required
The effectiveness of any deep learning model depends on the quantity and quality of the training data. Of course, not everyone has this amount of data available. This high data abundance dependence of deep learning systems is a limitation. It also leads to unfortunate incidents such as the British police program's inability to distinguish between sand dunes and bare dunes.
2. Not understanding the context
Another disadvantage of deep learning is that it cannot adapt to changing conditions.
For example, a deep learning model trained to play one game can beat the current human champion. However, suggesting him a different game and the same set of instructions for the model does not guarantee victory.
The need to retrain deep learning models every time every context changes can be seen as a constraint in a period of rapid growth.
Researchers and scientists have tried to point out other limitations of deep learning. Some come from outright skepticism, while others represent genuine constructive criticism. Steady progress is being made to improve deep learning and its effectiveness.
Conclusion
Deep learning has already become an integral part of our daily life and the services we use. In the future, it is likely that deep learning will become more widely accepted and diffused. Its utility in many fields and industries demonstrates the potential of the DL model. The forecast shows the phenomenal growth of the Deep Learning market over the next few years.
Now is a great time for businesses to dive into the world of AI and DL. It is also an encouraging picture for companies and industries that will benefit from deep learning.